The AI Opportunities Action Plan, a set of recommendations for the UK government developed by tech entrepreneur Matt Clifford, and the government’s 50 point response together aim to establish the nation as a global AI superpower, leveraging advancements to drive economic growth, enhance public services, and foster innovation. However, a critical question underpins this goal: can the UK’s energy infrastructure, already under strain, sustain the demands of AI-powered data centers, especially as the UK government recently announced that data centers are now recognised as Critical National Infrastructure (CNI)? Moreover, achieving sovereign AI, which ensures data integrity and security within national borders, adds another layer of complexity—and opportunity.
Context of the announcement: sovereign AI, a national priority
At the core of the AI Opportunities Action Plan is the development of a sovereign AI compute environment, which will require a sovereign AI ecosystem supported by a national cloud infrastructure that will securely store, process, and analyze sensitive data within the UK, aligning with national security, privacy, and data sovereignty requirements.
AI workloads and their energy dynamics
AI and High Performance Compute (HPC) workloads, especially those for large language models (LLMs) or advanced analytics, demand high-performance AI computing resources, which in turn require different energy demands. These workloads can be broadly classified into:
- Training: A computationally intensive process that requires stable, high-energy inputs over extended periods of time. Training is a schedulable process and can be matched to periods of high renewable energy production.
- Inference: A more variable and dynamic workload that involves executing AI models for real-time tasks like image recognition or natural language processing, where the consumption of power will vary for inference processes depending on the number of users and queries executed. Inferencing requires much less computation than model training, and GPUs or CPUs can be used.
Renewable energy: a double-edged sword
Renewable energy (RE) is central to the UK’s strategy to achieve electricity demand sustainably. However, renewable energy resources like wind power, while environmentally sustainable, typically fluctuate and are considered ‘intermittent’ energy resources, presenting a challenge for data centers, which require consistent and reliable power.
The new AI Energy Council, outlined in the government’s response to the AI Opportunities Plan, will play a critical role in exploring innovative energy solutions, including the potential for Small Modular (Nuclear) Reactors (SMRs), to address this gap. This will become the highest priority in days to come as data center energy predictions ‘pre’ AI production were climbing (see figure 1), but now are expedited by AI energy consumption (see figure 2), and expected to drive 160% increase in data center power demand according to Goldman Sachs (2024)
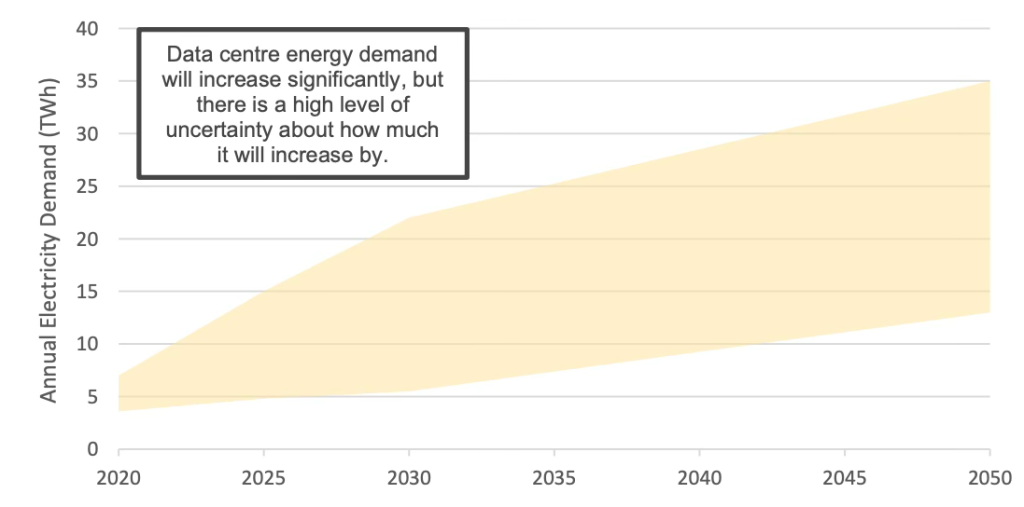
Figure 1: National Grid ESO report (2022)
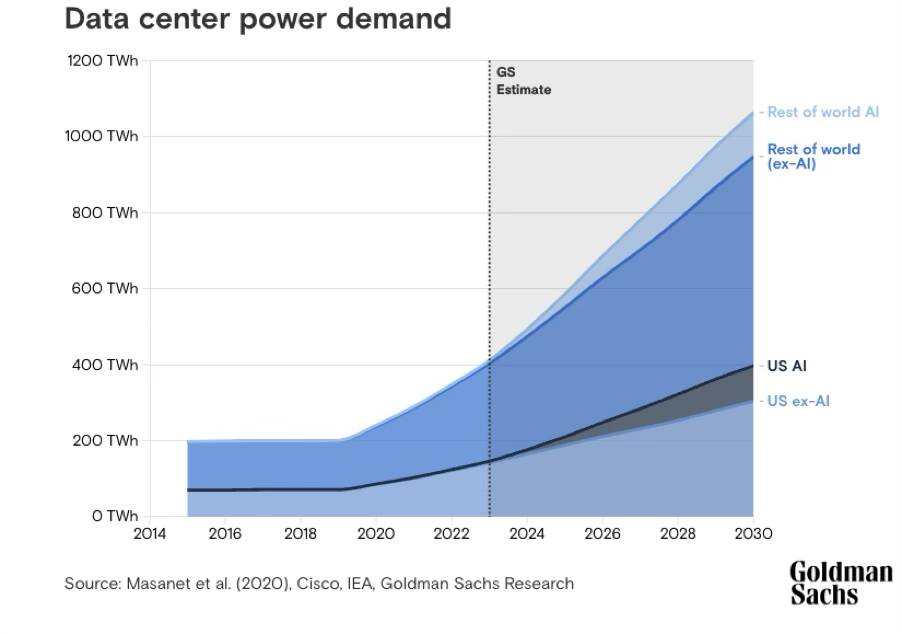
Figure 2: AI Energy research 2024
This unexpected growth in energy demand and consumption exceeds energy efficiency gains as wider use of AI becomes mainstream. For example, ChatGPT surpassed 100 million users in just two months of launch in 2023. In 2025, it is closing in on 200 million users, with approximately 464 million visits per month (in Nov 2024). The increasing usage of such solutions has a negative impact on energy utilization as AI models are more energy intensive in their computation. For example:
A single ChatGPT query requires 2.9 watt-hours of electricity, compared with 0.3 watt-hours for a Google search, according to the International Energy Agency.
https://www.goldmansachs.com/insights/articles/AI-poised-to-drive-160-increase-in-power-demand
Learning from global examples
According to the UK NESO report, countries like Ireland and the Netherlands have already faced energy challenges related to data center growth. Ireland, for instance, imposed restrictions on new data centers to prioritize RE use. Similarly, Amsterdam temporarily halted data center developments to address land and power constraints. Also, in turn, the UK has had to pull back on housing commitments to avoid energy shortages for essential needs such as residential power supply.
Aligning AI workloads with Renewable Energy
The UK is a RE leader and has a large amount of renewable energy resources, including wind, solar, biomass, and hydropower. In 2023, renewable energy sources accounted for 36.1% of the electricity generated in the UK, of which 29.4% came from wind, which is very variable. However, renewable energy alone for the AI Opportunities Plan will not be enough. AI data centers will need to adopt intelligent cooling (typically AI-driven, e.g.,Deep Cooling, Intel and Quarkdata), workload scheduling and resource management strategies to not exceed the available and intermittent energy demands of AI. Key approaches could include:
- Flexible scheduling: Leveraging periods of peak renewable energy (e.g., wind) generation for AI training tasks while aligning inference workloads with times of lower energy demand.
- Dynamic resource allocation: Using intelligent platforms to dynamically allocate compute resources based on energy availability and workload priorities.
- Energy-Aware Optimization & intelligently optimize datacenter cooling: Employing intelligent energy & cooling monitoring and data center power optimization tools, to align operations with renewable energy supply.
- Dynamic switchover: Managing data center demand, peaks and troughs with constant baseline supply from SMRs and complemented by, rather than reliant on, RE.
Broadcom VMware Cloud Service Providers: enabling efficiency and sovereignty with collaboration and ecosystem
Broadcom’s VMware Cloud Service Provider capabilities make them a natural choice for more energy efficient AI data centers operating within a sovereign cloud infrastructure. Key advantages include:
- Energy-optimized data centers:
- VMware Cloud Service Providers utilize varying energy efficient data centers designs and solutions to minimize energy costs, optimize environmental-social-governance (ESG) strategies and help support companies to address upcoming regulatory requirements, like the UK Sustainability Reporting Standards (UK SRS).
- VMware Cloud Service Providers typically purchase RE and utilize solutions within their data centers to analyse and predict energy consumption behaviour, dynamically adjust parameters to meet demands of power loads and cooling.
- Resource efficiency:
- VMware Cloud Service Providers enable precise resource allocation and utilization, ensuring that AI workloads consume only the necessary energy. This minimizes wastage, especially during periods of limited renewable energy availability.
- VMware Cloud Foundation, a core technology for VMware Cloud Service Providers, provides benefits like Distributed Resource Scheduler (DRS) balances workloads across available infrastructure, aligning with energy-responsive computing needs. Although an established power saving technology (up to 40% energy saving in some cases), DRS is a VMware product only feature, not available in other hypervisors’ products.
- AI requires high levels of compute resources and typically specialized hardware like GPUs. These resources compute high levels of parallel transactions, allowing extreme capacity required for AI Models and applications. VMware offers multi-tenanted GPU virtualization and graphics virtualization solutions, effectively consolidating resource utilization and reducing the need for additional hardware and therefore energy. See this blog analysis.
- Sovereign Cloud solutions:
- VMware Cloud Service Providers delivering VMware Sovereign Cloud offer secure, localized data processing capabilities that comply with UK data governance regulations. Examples of UK providers include Redcentric & OVHCloud, both of which are G Cloud compliant and offer solutions that use energy efficient data centers and can help with climate impact reporting schemes like ESOS (Energy Savings Opportunity Scheme) and TCFD (Task Force on Climate-related Financial Disclosures) to measure and disclose energy consumption and climate-related impacts.
Digital sovereignty is one of OVHcloud’s major strengths, and the vendor can apply this to all levels of sovereignty (data, technical, and operations).’
OVHcloud named a Major European Public Cloud IaaS Player - IDC MarketScape 2024
- This ecosystem of partners and their VMware and non-VMware solutions ensures that AI workloads, data and operations are managed and processed within UK borders, maintaining compliance with national data sovereignty requirements.
- Scalability for hyper-scale and hybrid environments:
- VMware supports the deployment of data centers that can train large-scale AI models.
- VMware also facilitates hybrid cloud environments, enabling organizations to leverage on-premises resources alongside national cloud infrastructure for enhanced flexibility.
- Energy-responsive AI operations:
- VMware’s leading advanced monitoring/energy monitoring and automation tools allow data centers to adjust workload execution based on real-time energy availability, which can contribute to more environmentally sustainable operations.
It’s important to note there is no silver bullet for energy optimization in data centers. The combination of workload variations, hardware variations and infrastructure variations makes it nearly impossible to say what solution outperforms another.
The possible role of VMware Sovereign Cloud in AI leadership
Sovereign VMWare Cloud Service Providers could host the Action Plan’s proposed AI ‘Growth Zones’ and other data center infrastructures, ensuring secure, high-performance environments that meet government and enterprise requirements:
- Data Sovereignty: UK owned and operated providers ensure sensitive information is kept within UK borders.
- Compliance: Meeting regulatory requirements for the UK public sector and critical national infrastructure workloads.
- Operational Efficiency: Leveraging VMware Cloud Foundation to optimize resource usage, reduce energy consumption, and enable dynamic workload management.
Advanced resource management: optimizing GPU utilization for energy efficiency
Managing GPU resources effectively is essential for optimizing performance and minimizing energy consumption in data centers, particularly as the UK positions itself for AI and digital growth. GPUs are significantly more energy-efficient than CPUs for AI inference tasks—studies show up to 42x greater efficiency—but their increasing cost and energy intensity make strategic allocation crucial. Given the complexity of GPU scenarios, which vary depending on applications, query types, and user volume, ensuring these powerful resources are fully utilized and not left idle is a top priority for reducing environmental impact and maximizing return on investment. Effective GPU optimization strategies, supported by platforms like VMware Clouds, include dynamic sharing and partitioning techniques, enabling better resource allocation, minimizing wastage, and supporting data centers transitioning to renewable energy sources.
Key GPU resource allocation strategies
- Virtual GPUs (vGPU):
- vGPU technology enables multiple workloads to run within containerized environments by sharing a single physical GPU across virtual machines (VMs).
- This approach allows for greater flexibility and scalability, ensuring that GPU resources are allocated dynamically based on workload demand while minimizing underutilization
- Multi-Instance GPUs (MIG):
- MIG technology partitions a physical GPU into multiple, isolated instances at the hardware level.
- This enables multiple applications or users to share a single GPU without compromising performance isolation or security. By reducing idle time and ensuring high utilization rates, MIG enhances overall energy efficiency and lowers operational costs.
- GPU Time-Slicing:
- Time-slicing divides GPU processing power into discrete time segments, allowing multiple workloads or VMs to share a single GPU.
- This ensures fair allocation of GPU resources, supports concurrent execution of tasks, and maximizes resource utilization by eliminating bottlenecks caused by workload imbalances.
Making the right platform choice
When choosing between differing vendors for CPU and GPU resource management in these new datacenters the following should be factors of consideration:
- Workload requirements: Not every workload is going to be an AI workload, and thus, the platform must be able to support enterprise-grade workloads requiring advanced CPU and GPU virtualization and hybrid cloud support.
- Budget constraints: The platform should feature tried and tested robust capabilities at a cost-effective budget.
- Ease of Management: Ease of use and centralized management, will be crucial factors to monitor and operate these data centers efficiently.
- Scalability: Seamless scalability for hybrid and multi-cloud environments, with open-source ecosystem support and diverse application portfolios are necessary to ensure longevity and future repurposing for alternative tasks/applications.
By aligning platform capabilities with organizational goals, data centers can optimize GPU resource allocation, reduce energy consumption, and support the growing demand for AI workloads.
A vision for energy-aware Sovereign AI in the UK
As the UK strives to position itself as an AI superpower, integrating sovereign cloud solutions with energy-aware technologies like VMware Cloud Foundation provides a clear path forward. This approach not only addresses the energy challenges associated with AI workloads but also ensures the security, scalability, and compliance necessary for a thriving national AI ecosystem.
To achieve this, stakeholders must collaborate on:
- Renewable Energy Alignment: Optimizing AI workload scheduling to maximize the use of wind and other renewable energy sources.
- Sovereign Platform Infrastructure Investment: Expanding Sovereign Cloud and other national cloud initiatives to support ‘hyper-scale’ volumes and hybrid deployments.
- Advanced Resource Management: Leveraging VMware’s Cloud capabilities for efficient, energy-responsive operations.
Path forward: balancing growth and environmental sustainability
To align the AI goals with the realities of the energy grid, the UK must develop a path forward considering:
- Energy Efficiency: Encourage innovative technologies to reduce energy consumption in data centers, such as liquid cooling and AI-driven energy optimization.
- Grid and Consumption Modernization: To support the scaling of data centers in line with economic growth, it is crucial to accelerate grid modernization and expedite the time to deliver new power grid connections for data centers and increasing capacity (40% of new grid connection agreements sold feature connection times extending into the 2030s) .
- Diversified Energy Mix: Invest in both renewable energy and complementary solutions like Small Modular Reactors (SMRs) to ensure a stable supply and utilize data center energy monitoring and allocation modelling.
- Regulatory Frameworks: Introduce policies that incentivize energy efficient and environmentally sustainable data centers while managing growth to avoid overwhelming the grid.
- Collaboration with Industry: Engage private-sector and regional cloud provider stakeholders to co-develop environmentally sustainable energy strategies for data centers. Additionally, collaboration between Ofgem and the Environment Agency is essential to address the challenges of energy consumption and modern water cooling systems and usage in data center operations.
By aligning its AI goals with more environmentally sustainable and sovereign solutions, including Sovereign VMware Cloud Service Providers, the UK can lead the way in shaping a future where technology, energy efficiency and security go hand in hand.