In this blink-and-you-miss-it tech landscape, a recurring challenge stands out: while we’re seeing incredible new AI applications emerge, especially at the edge, there’s often a gap in understanding the network infrastructure required to support them. AI-driven applications are disrupting long-established upstream/downstream edge traffic ratios, their traffic is encrypted and therefore hard to identify for analysis and prioritization, and agentic AI workloads are sensitive to latency and dropped packets. In VeloCloud’s State of The Enterprise Edge report, many organizations stated they are initiating “AI networking” infrastructure refresh projects to better support these unique AI workload requirements outside the datacenter.
VeloRAIN is an AI networking architecture that enhances the performance, security, and scalability of distributed AI workloads beyond traditional data centers. Short for VeloCloud Robust Artificial Intelligence Networking, VeloRAIN builds on the leading VeloCloud SD-WAN platform to optimize AI workloads across distributed enterprise networks. It enhances VeloCloud Dynamic Multipath Optimization™ (DMPO) with AI, and introduces Dynamic Application-Based Slicing (DABS) to assure quality of experience (QoE) across multiple networks. With VeloRAIN, organizations can use AI apps more easily with visibility, app prioritization, and automation that continually improves QoE.
VeloRAIN was born from data-driven insights
As the leading SD-WAN provider, VeloCloud has more than 18,000 customers including telecom operators, service providers, and enterprises. Over the past year, our extensive network — more than half a million deployed VeloCloud SD-WAN Edges — has given us unique insights into network and application performance across every vertical.
Each VeloCloud Edge functions as a sensor within our customers’ environments, offering real-time data on application usage and WAN performance. When we observe this (anonymized) data, we see a significant surge in the adoption of AI applications. The rise began with innovations like video inferencing, chatbots, and co-pilots and has since expanded to include agentic AI technologies and retrieval augmented generation (RAG)-based systems, even extending into consumer networks.
Why does AI traffic need a different network architecture?
In VeloCloud’s ongoing analysis of AI applications, we uncovered a critical insight: the nature of these apps is fundamentally altering edge networking. AI applications are distinct not only in their operational patterns but also in how they use network resources. They are bursty, highly sensitive to latency, and often operate on a peer-to-peer basis.
In the past, the primary traffic direction for edge sites was downstream and usage swings were addressable via QoS and policy. But AI applications can radically change the upstream/downstream balance and make WAN traffic unpredictable. Because AI traffic is encrypted, it can’t be distinguished from other flows and therefore can’t be optimized.
Consider the Ray-Ban AI glasses from Meta. They primarily send video data upstream, while audio feedback remains downstream. In a typical network, video streaming traffic is heavily skewed towards the downstream, often at ratios of 99 to 1. However, the glasses send upstream traffic in the same ratio. AI network traffic is also bursty and unpredictable. For example, GenAI interactions typically involve high traffic when the client uploads a large request, a pause as the server processes and prepares a response, and then a large response is sent back to the client.
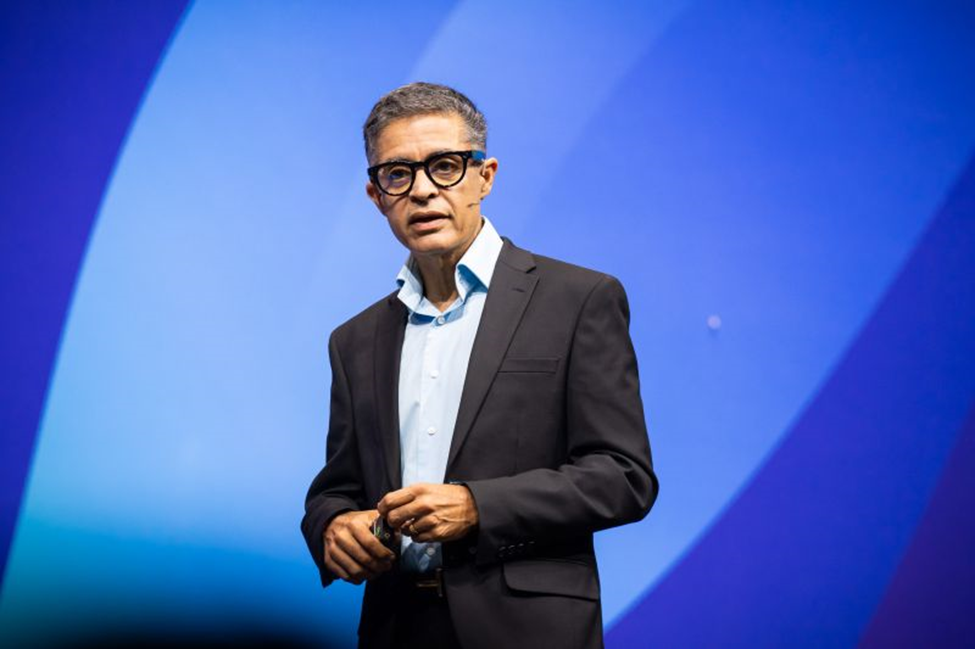
The challenge extends beyond developing superior algorithms for network management outside the data center. It also involves adapting to the unique requirements of these AI-driven applications through an intelligent software layer. This layer must not only understand the applications’ needs but also dynamically adjust network resources to prioritize critical applications, ensuring optimal quality of service while deprioritizing less critical traffic.
The VeloRAIN architecture enhances VeloCloud’s DMPO network intelligence to accommodate these evolving demands efficiently. This ensures that enterprises can use AI capabilities effectively, without compromising on network performance or user experience.
The three pillars of VeloRAIN
Three critical aspects of the VeloRAIN architecture set it apart in optimizing AI applications.
- AI-driven application profiling: VeloRAIN identifies and prioritizes applications with new intelligent capabilities. By identifying applications accurately through an enhanced machine learning (ML) system, VeloRAIN ensures that each AI and non-AI app receives appropriate network resources. VeloRAIN will also be able to identify encrypted application traffic that was previously unreadable for network optimization solutions. Organizations will be able to tailor network performance to meet the specific needs of or critical applications.
- AI-based network optimization: VeloRAIN introduces Dynamic Application-Based Slicing (DABS), an innovative approach focusing on the application layer instead of traditional network-based slicing. This assures quality of experience (QoE) per application across multiple disparate underlying networks, whether they support network layer slicing or not. AI workloads receive the necessary bandwidth and low latency required for optimal performance, while less critical applications can be de-prioritized. DABS also includes user profiles to prioritize traffic based on a user’s identity and attributes, helping ensure that key users receive the QoE they need.
AI also augments VeloCloud’s DMPO (AI DMPO). Feeding packet capture data into an LLM, and then training that LLM to perform packet capture data, will allow end users to ask natural-language questions such as “Why is the network running slowly?” and receive an immediate response. - AIOps with real-time data: With data from its vast deployment base, VeloRAIN employs AI to automate network operations (AIOps), using anonymized real-time data to dynamically adjust policies. For example, in retail stores, vision applications analyzing in-store behavior could benefit from real-time policy adjustments, ensuring seamless experiences and high-value customer retention. This dynamic approach allows enterprises to adjust network configurations on-the-fly, ensuring the best possible experience for users and applications.
Enabling AI traffic with dynamic channel prediction and adaptive slicing
Understanding the nature of the network is critical to respond to AI traffic. Networks can vary widely, from MPLS and fiber to broadband connections. Satellite and fixed wireless access (FWA) have evolved from failover options to primary links as they become more reliable and less expensive. To accommodate this variability, VeloRAIN applies machine learning to perform channel estimation for networks, including satellite, 4G, and 5G. By accurately predicting channel conditions, VeloRAIN can adaptively manage bandwidth, latency, and jitter, crucial for supporting asymmetric traffic patterns of AI-driven applications.
With the explosion of AI-driven, peer-to-peer applications, VeloCloud’s approach to application-based slicing allows for a focused QoE at the application layer. This method enables network administrators to ensure that high-value AI applications receive priority over less critical applications, optimizing both resource allocation and user experience.
High scalability for agentic AI models
Agentic AI systems use multiple agents to collaborate to answer users’ questions, and it has significantly increased peer-to-peer traffic. In these complex interactions, a human query initiates a sequence of communications. A supervisory agent receives the question and coordinates with multiple other agents. These agents then engage in a collaborative, peer-to-peer dialogue across the distributed enterprise network.
VeloRAIN plays a pivotal role in managing this intricate network activity. By establishing segmentation—a virtual construct layered over physical network infrastructures—VeloRAIN can effectively map and oversee the peer-to-peer interactions among agents. This approach not only enhances security by isolating agent-to-agent traffic from other network activities, but also ensures superior quality of service.
The growing popularity of agentic AI models underscores the need for robust network solutions. As specialists in intelligent network overlay technologies, VeloCloud is refining our segmentation offerings to better support these models’ unique demands. This proactive adaptation ensures that our network infrastructure not only meets current demands but also anticipates future networking needs, providing our customers with a secure, efficient, and scalable networking environment.
Why is VeloRAIN the right choice for AI networking?
In recent years, there has been a perception that the advanced capabilities of SD-WAN might not be necessary as enterprises move increasingly towards cloud-based models, relying merely on basic cloud on-ramps. However, this view overlooks important requirements of today’s applications —sophisticated network solutions like segmentation, controlled access, and a robust, intelligent overlay across multiple disparate links. These are capabilities that VeloCloud SD-WAN provides.
VeloCloud SD-WAN is essential for supporting the peer-to-peer mesh architectures that AI applications require. But we recognize that to truly enhance application performance and network efficiency, additional capabilities are necessary beyond the standard SD-WAN offerings. VeloCloud has been actively developing further innovations such as AI DMPO, last-mile optimization, link aggregation, and advanced application identification and profiling. These enhancements are designed to add significant value over our existing services, ensuring that enterprise workloads not only run smoothly but also optimally.
The growth in AI applications underscores the importance of responsive and adaptive network technologies. The VeloRAIN architecture is at the heart of Broadcom’s strategy, designed to not only recognize changes within network traffic but also to respond dynamically to these changes. VeloRAIN will underpin all VeloCloud family solutions including VeloCloud SASE, secured by Symantec and VeloCloud SD-Access, for the best performance and enhanced user experiences across our customers’ networks.
VeloCloud and Broadcom understand the evolving needs of modern enterprises, especially as they navigate the complexities of cloud integration and application management. Our SD-WAN expertise positions us uniquely to address these challenges, making us the right partner for businesses that are getting their network infrastructure ready for the AI future.